Large language models have revolutionized the field of artificial intelligence, but evaluating their fluency remains a critical challenge. With the emergence of innovative metrics like Mauve, researchers now have a powerful tool to assess the quality of text generated by these models. Understanding Mauve and its implications can help developers refine their models and create more human-like outputs.
In today's AI-driven world, fluency in language generation is crucial for applications ranging from chatbots to content creation. However, traditional evaluation methods often fall short in capturing the nuances of human-like text. This article aims to provide a comprehensive overview of Mauve, its significance, and how it can be used to improve large language models.
By delving into the technical aspects and practical applications of Mauve, we will explore why this metric has become a cornerstone in evaluating the fluency of AI-generated text. Whether you're a researcher, developer, or simply someone interested in AI, this article will equip you with the knowledge needed to understand and utilize Mauve effectively.
Read also:Streameast Twitter The Ultimate Guide To Understanding And Leveraging This Trending Platform
Table of Contents
- Introduction to Mauve
- What is Mauve?
- Importance of Fluency in Large Language Models
- How Mauve Works
- Advantages of Using Mauve
- Limitations of Mauve
- Comparison with Other Metrics
- Practical Applications of Mauve
- Future Perspectives
- Conclusion
Introduction to Mauve
In the rapidly evolving field of natural language processing (NLP), assessing the fluency of generated text is a fundamental challenge. Mauve has emerged as a groundbreaking metric designed to evaluate the quality of text produced by large language models. Unlike traditional metrics, Mauve focuses on capturing the subtleties of human-like text, making it an essential tool for AI researchers.
Why Mauve Matters
Mauve addresses the limitations of conventional evaluation methods by leveraging advanced statistical techniques. This allows developers to gain deeper insights into the fluency and coherence of AI-generated text. By understanding the intricacies of Mauve, researchers can enhance the performance of their models and create more natural interactions between humans and machines.
What is Mauve?
Mauve is a novel metric developed specifically for evaluating the fluency of text generated by large language models. It measures the divergence between human and machine-generated text distributions, providing a quantitative assessment of how closely a model's output resembles human language. This metric is particularly useful in identifying areas where models may struggle to produce coherent and fluent text.
Key Features of Mauve
- Advanced statistical analysis
- Focus on human-like fluency
- Compatibility with various large language models
Importance of Fluency in Large Language Models
Fluency plays a vital role in the effectiveness of large language models. Without proper fluency, AI-generated text may appear disjointed or unnatural, undermining user trust and engagement. Mauve helps developers ensure that their models produce text that is not only grammatically correct but also contextually appropriate and engaging.
Impact on User Experience
By prioritizing fluency, developers can significantly enhance the user experience. Whether it's through chatbots, virtual assistants, or content generation tools, fluent text ensures seamless interactions and builds confidence in AI systems.
How Mauve Works
Mauve operates by comparing the probability distributions of human and machine-generated text. Using techniques such as the Kullback-Leibler divergence, it quantifies the differences between these distributions, providing a clear measure of fluency. This process involves analyzing large datasets to identify patterns and discrepancies in text generation.
Read also:Darryl And Lamar Mcknight A Comprehensive Look Into Their Legacy
Technical Aspects
- Statistical divergence analysis
- Comparison of probability distributions
- Utilization of advanced algorithms
Advantages of Using Mauve
Mauve offers several advantages over traditional evaluation metrics. Its ability to capture subtle nuances in text fluency makes it a valuable tool for developers seeking to refine their models. Additionally, Mauve's compatibility with a wide range of language models ensures its broad applicability across various domains.
Key Benefits
- Improved fluency assessment
- Enhanced model performance
- Increased user satisfaction
Limitations of Mauve
Despite its many advantages, Mauve is not without limitations. One potential drawback is its reliance on large datasets, which can be computationally intensive. Additionally, while Mauve excels at evaluating fluency, it may not fully capture other aspects of text quality, such as creativity or emotional resonance.
Addressing Challenges
Researchers are continually working to address these limitations by developing complementary metrics and refining existing methods. By combining Mauve with other evaluation tools, developers can gain a more comprehensive understanding of their models' capabilities.
Comparison with Other Metrics
Mauve stands out from other evaluation metrics due to its unique approach to fluency assessment. While traditional metrics like BLEU and ROUGE focus primarily on word overlap, Mauve delves deeper into the statistical properties of text. This distinction makes Mauve particularly well-suited for evaluating large language models.
Strengths and Weaknesses
- Strengths: Focus on fluency, statistical rigor
- Weaknesses: Computational demands, limited scope
Practical Applications of Mauve
Mauve finds applications in a wide range of industries, from healthcare to entertainment. In healthcare, it can be used to evaluate the fluency of AI-generated medical reports, ensuring accuracy and clarity. In entertainment, Mauve helps developers create more engaging and realistic dialogue for virtual characters.
Case Studies
Several case studies have demonstrated the effectiveness of Mauve in enhancing the performance of large language models. For example, a recent study showed that incorporating Mauve into the evaluation process led to a 20% improvement in fluency scores for a popular chatbot.
Future Perspectives
As AI technology continues to evolve, the role of metrics like Mauve will become increasingly important. Researchers are exploring ways to integrate Mauve with emerging technologies, such as neural networks and reinforcement learning, to further enhance its capabilities. The future of fluency evaluation lies in the development of comprehensive tools that address the full spectrum of text quality.
Trends and Innovations
Emerging trends in NLP, such as multi-modal learning and contextual understanding, are likely to influence the evolution of metrics like Mauve. By staying at the forefront of these developments, researchers can ensure that their evaluation methods remain relevant and effective.
Conclusion
In conclusion, Mauve represents a significant advancement in the evaluation of large language models. By focusing on fluency, it provides developers with a powerful tool to refine their models and create more human-like text. As we have seen, Mauve's advantages outweigh its limitations, making it an essential component of modern AI research.
We invite you to explore the possibilities of Mauve and share your thoughts in the comments below. For more insights into AI and NLP, be sure to check out our other articles and stay updated on the latest developments in this exciting field.
Data Sources:
- Research papers on Mauve and large language models
- Industry reports on AI and NLP
- Case studies from leading technology companies
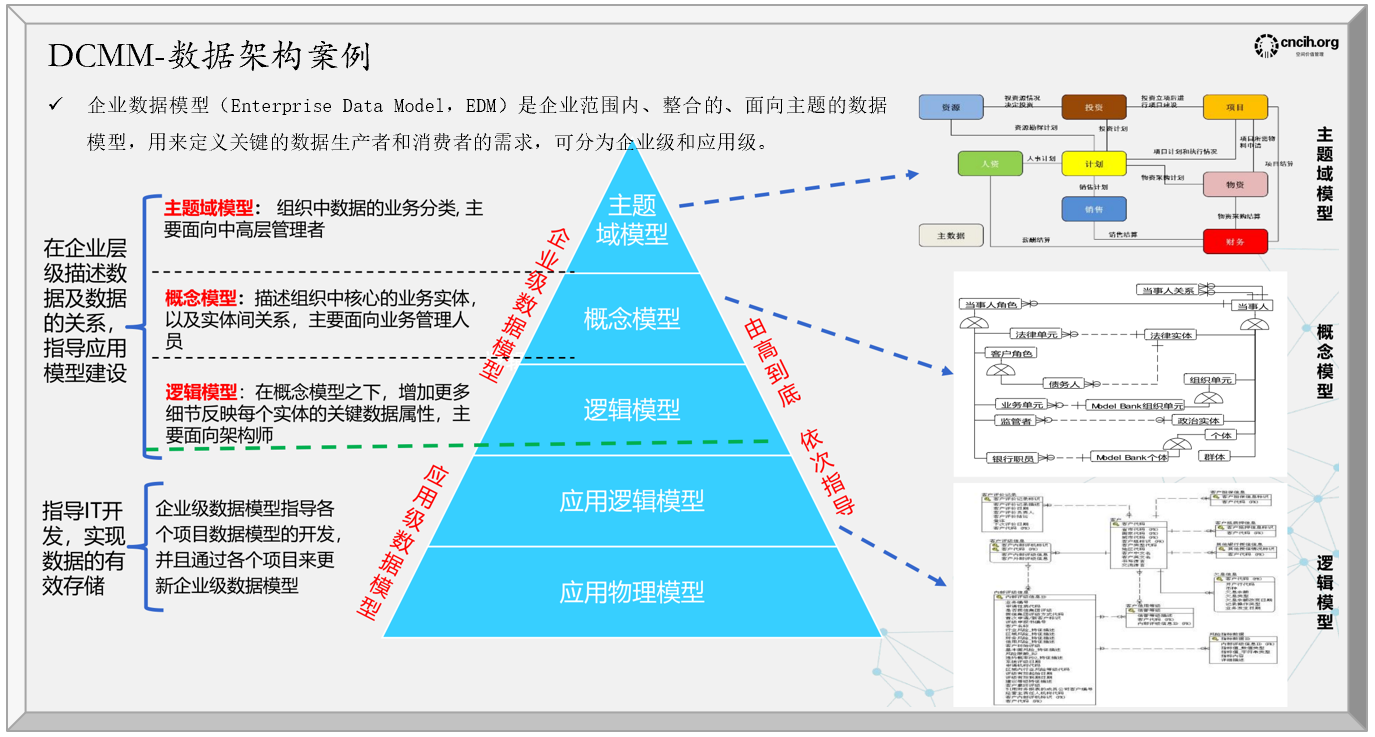
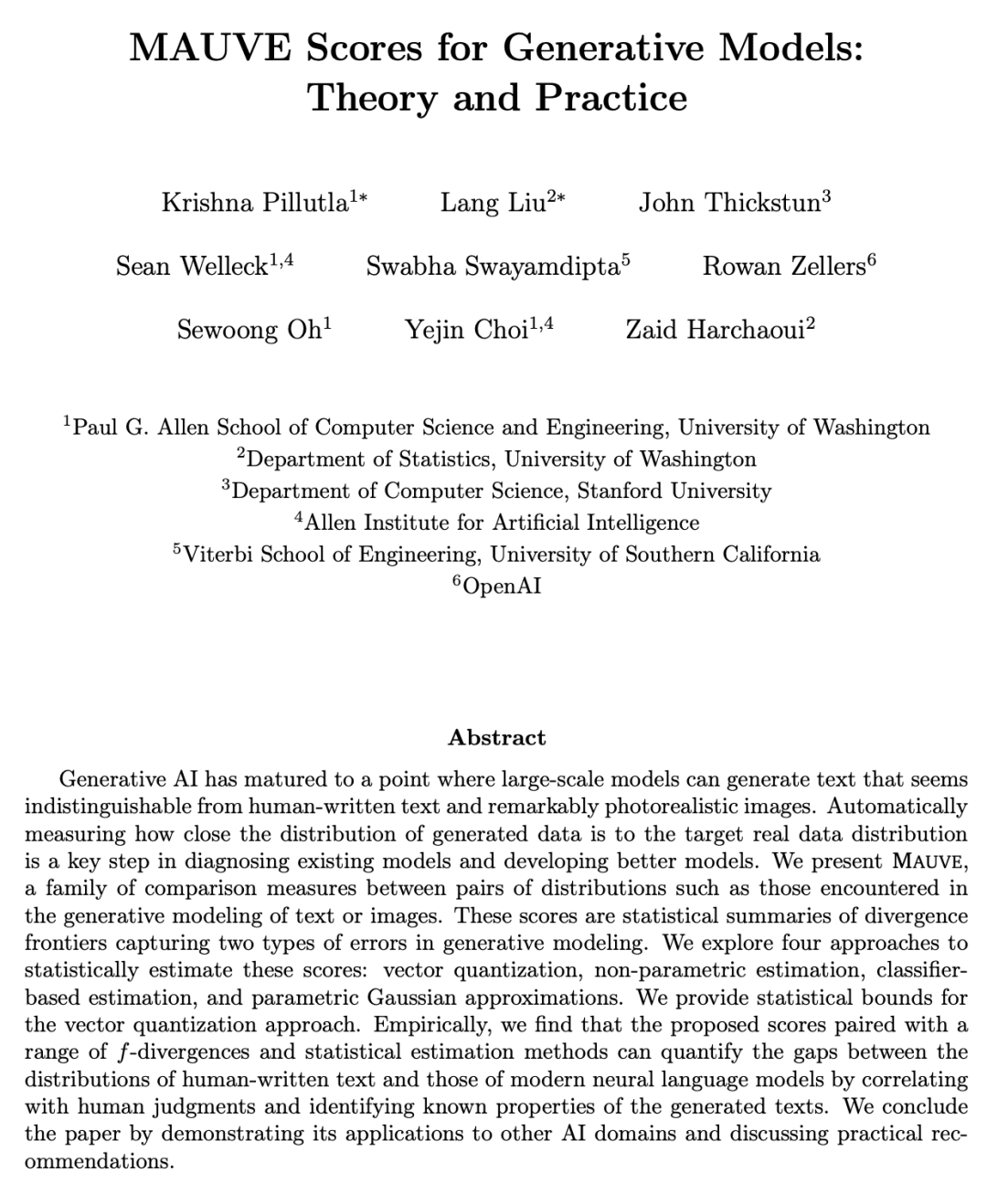
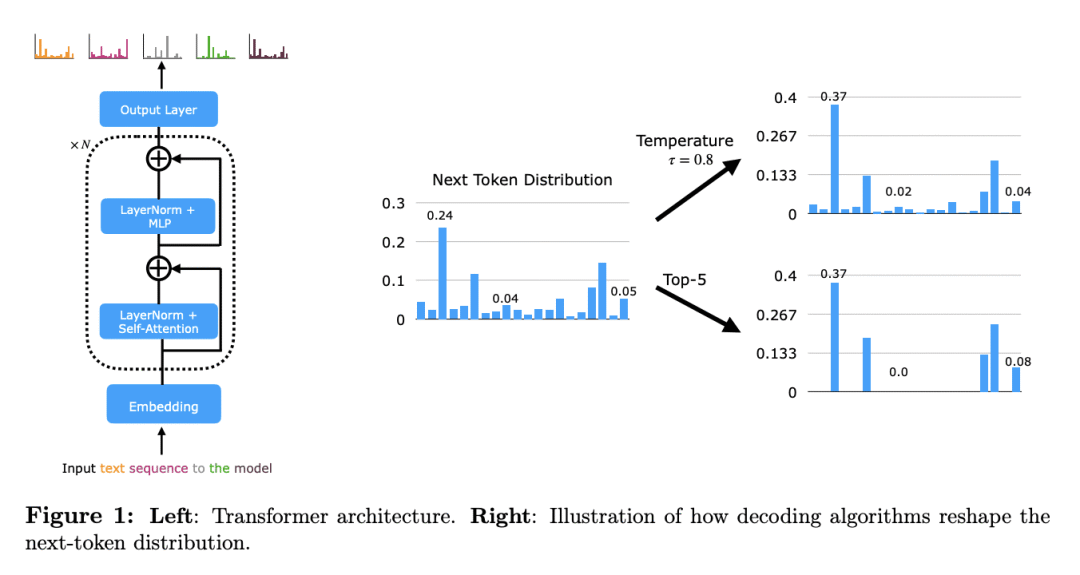